Startups know the pain: your team builds an MVP with a promising ML model, but getting that model into production takes weeks (or months) of patchwork scripts and late-night debugging. Monitoring breaks. Retraining is ad hoc. You’re forced to choose: slow down, or risk failure in the wild. That’s where Samey.ai steps up—a modern MLOps platform promising to automate, simplify, and supercharge your machine learning lifecycle.
But is Samey.ai truly startup-friendly, or just more “enterprisey” overhead? In this practical founder’s guide, we’ll break down what Samey offers, how it fits into lean workflows, and whether it really saves time, money, and founder sanity. From features and pricing to side-by-side alternatives, you’ll get the full scoop—mentor-style, no fluff. Ready to level up your MLOps? Let’s go. 🚀
Samey.ai Overview: Why Founders Pay Attention 👀
Samey.ai was born from the trenches—by ML engineers tired of duct-taping Jupyter notebooks to cloud scripts every time a product needed to scale. The pitch? A single platform to track, deploy, monitor, and orchestrate ML models with less technical friction. Founders love it for two core reasons:
- Focus on shipping, not wrangling ops: No more sending your one ML hire down DevOps rabbit holes. Samey.ai lets your team automate versioning, streamline experiments, and fast-track deployment—all from one clean UI or API. ✔️
- Growth without growing pains: Whether you’re validating MVPs or scaling to handle thousands of users, Samey promises self-serve infrastructure that adapts as you move from pre-seed to Series A. Think less firefighting, more feature building. 🔥
Most early-stage teams drop Samey into the pipeline after their first “It works on my laptop, but not in prod!” disaster. If you’re tired of babysitting cron jobs and praying to the gods of YAML, here’s what matters.
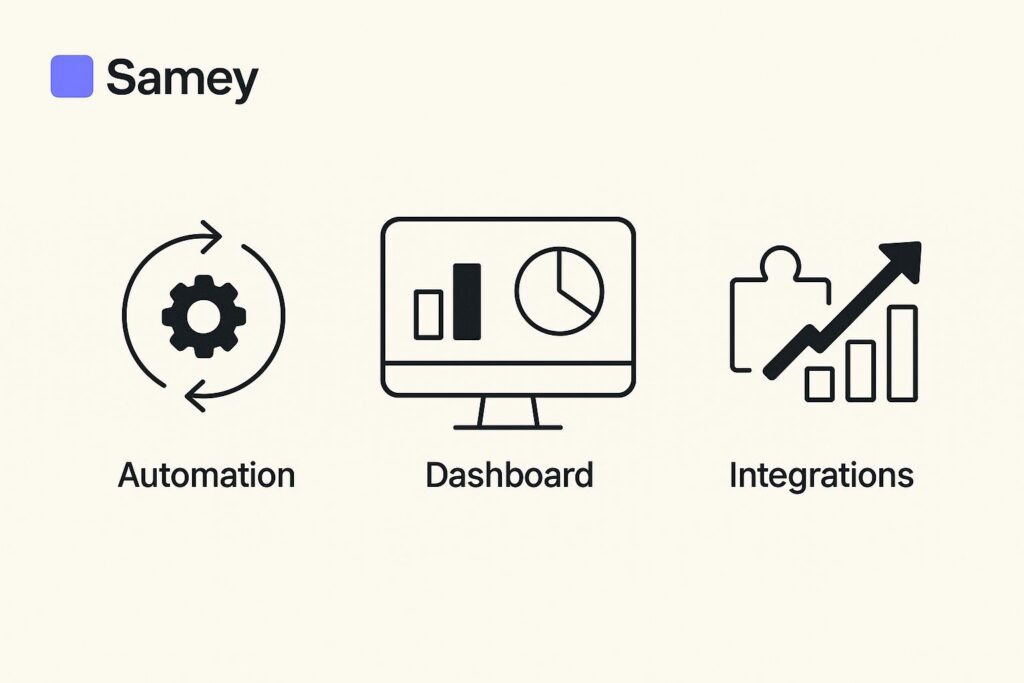
Key Features of Samey.ai
Let’s cut to the features founders actually use and care about:
⚡ One-Click Model Deployment
Spin up prediction endpoints from your notebook or CLI in seconds. No scary Kubernetes configs, no DevOps degree required.
🔄 Experiment Tracking & Reproducibility
Every run, hyperparameter, and dataset gets auto-versioned and visualized—so you don’t lose results when your laptop dies or an engineer leaves.
👀 Model Monitoring & Drift Alerts
Out-of-the-box dashboards alert you on data drift, prediction anomalies, or degraded accuracy. Stay ahead of silent failures (before your first paying customer emails).
🤝 Team Collaboration & Access Controls
Create shared workspaces, role-based project permissions, and code reviews—critical for async teams and regulated industries.
🔌 Built-in Cloud Integrations
Native connectors to AWS, GCP, Azure, Snowflake—plus APIs/webhooks for Slack, DataDog, and linear workflows (no more begging devs for custom hooks).
📦 Automated Retraining Pipelines
Schedule retrains on new data, pipe outputs to your favorite BI tool, and get Slack alerts if anything fails. Perfect for scrappy, fast iteration loops.
🧑💻 API-First Modular Design
Everything is accessible via a REST API or Python SDK—so you can integrate with custom tools or automate repetitive tasks.
🍃 Usage-Based Pricing
Pay for what you use: experiments run, storage, API calls. Perfect for startups with spiky or unpredictable needs.
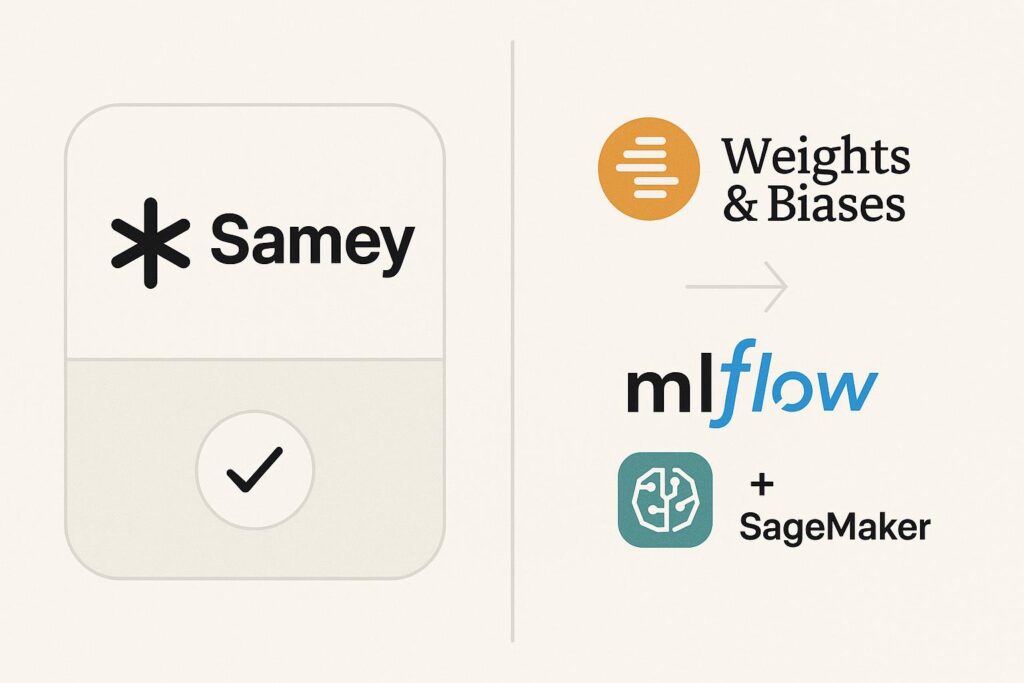
Samey.ai Pros and Cons
Here’s the honest founder’s-eye view:
👍 Pros:
- Rapid time-to-production for ML models, boosting your shipping cadence.
- Minimal setup and learning curve—great for teams without full-time infra/devops.
- Strong collaboration tools to keep remote, async teams aligned.
- Flexible integrations (cloud, CI/CD, notification tools) with no DIY glue code.
- Usage-based pricing prevents overpaying during your ramen-profitable phase.
- Alerts and dashboards that keep you off “fire drill” duty.
👎 Cons:
- Enterprise-y features gated to higher tiers (advanced RBAC, custom SLAs).
- Storage/API costs can scale fast if you don’t monitor usage.
- Fewer bleeding-edge ML features than DIY/JAX or open-source stacks.
- Less control than rolling your own with open tools (e.g., custom Kubernetes).
- Learning curve if migrating existing in-house infra.
Considering for Startups ✔️
Picking an MLOps platform isn’t like choosing a coffee subscription—it’s core to shipping products. Use this checklist to see if Samey makes founder sense for your stage:
- 📊 How complex are your ML workflows?
If you’re just training scikit-learn in a notebook, you may not need all the bells and whistles (yet). More complex pipelines benefit more from automation. - 🧠 Do your founders/team grok DevOps?
No infra wizards? Samey reduces the “weekend DevOps bootcamp” stress. - 💰 What’s your real MLOps budget?
Usage-based tiers are great, but surprise costs can burn cash. Stay vigilant—monitor usage or pick a capped plan. - 🔗 Does it integrate with your current stack?
Startup workflows can be fragile. Make sure Samey plays well with your cloud, codebase, and data tools. - ⚙️ How often do you retrain or update models?
Frequent iterations (think: product tweaks, new data weekly)? Samey automates retraining and reduces engineering overhead. - 🌟 Are you pre- or post-product-market fit?
Early MVPs may not need full-scale ops. But if you’re scaling users, stability and monitoring matter. - 👥 How many engineers will use it?
Solo data scientist? Small founding team? Choose plans that don’t force multi-seat minimum pricing. - 🚀 Will you need to scale fast or hit compliance?
If you anticipate big customer jumps, HIPAA, or SOC2, roadmap your infra choices to avoid future rework.
Samey.ai Plans and Pricing
Plan | Monthly Price (Annually Billed) | Usage Limit | Key Features |
---|---|---|---|
Starter | $0 | 3 concurrent runs, 2GB storage | Basic deployment, experiment tracking, community support |
Growth | $39 | 20 runs/mo, 20GB storage | Monitoring, retraining, team workspaces, priority support |
Scale | $159 | 100 runs/mo, 100GB storage | API access, RBAC, cloud integrations, Slack/Datadog hooks |
Enterprise | Custom | Unlimited | SOC2/HIPAA, onboarding, SLA, VPC deployment, advanced security |
Founder tips:
- The free Starter tier is genuinely usable for MVP/prototype work.
- Upgrades happen naturally—when you need more runs/storage or integrations.
- API usage and storage overages can add up; review before scaling experiments.
Samey.ai Startup Discount and Promo Info
Updating …
Comparing Samey.ai with Alternatives
Here’s how Samey stacks up against other popular MLOps platforms founders consider:
🧬 Feature | Samey.ai | Weights & Biases | MLflow + Sagemaker |
🏱 Free tier | Yes, usable | Yes, tracking only | Yes, very basic |
🚀 Model deployment | Instant, one-click | Manual, multi-step | Managed (Sagemaker only) |
📊 Monitoring | Drift, latency, errors | Customizable, some code | Basic (Sagemaker only) |
🔌 API Integrations | Python SDK, REST | Python CLI/API | Python API/SDK |
⚡ Usage pricing | Usage + per-feature | Mostly per seat, usage | Pay-as-you-go (AWS) |
🧬 Collaboration | Workspaces, RBAC | Project teams, RBAC | Siloed, complex perms |
🌟 Best for | Fast-moving startups | ML-heavy scaleups | DIY-savvy eng. teams |
Summary: Samey is the speediest for deploy-and-iterate, wins on out-of-the-box monitoring, and offers simpler, more predictable pricing for small teams.
FAQs
❓ Is it beginner-friendly?
Yes—onboarding is slick, and you can deploy models from the web UI or CLI with no DevOps experience. Minimal friction for first-time founders and data scientists.
❓ Are there hidden costs?
Most are transparent, but overages (runs, storage, API calls) can surprise if you scale fast or run a huge volume of experiments.
❓ Can I self-host Samey for compliance?
Only on the Enterprise plan—which is custom-priced and includes SOC2/HIPAA-ready deployments.
❓ What cloud platforms does Samey support?
AWS, GCP, Azure, and on-prem connectors. Plug-and-play for common startup stacks.
❓ Can my whole team collaborate?
Yes—all paid tiers support team workspaces, with access control and permissions.
❓ Does Samey integrate with Slack/DataDog/Zapier?
Natively on Scale and higher tiers. You’ll get hooks for alerts, monitoring, and workflow automation.
❓ What if we need feature X later?
Samey’s modular API means you usually don’t hit a “hard wall.” But ultra-custom ML infra (Kubernetes, GPU clusters) are better handled by DIY or enterprise platforms.
❓ What’s the support like?
Community and email for Starter/Growth. Priority/personal support on Scale/Enterprise.
Final Thoughts
For early-stage teams, Samey.ai is a powerful “ops-in-a-box” that can help you move from ML prototype to production-grade product—without letting MLOps complexity eat your lunch. 🌯
It’s built for shipping fast, collaborating in tiny teams, and integrating with the typical startup stack. If your priorities are MVP speed, not building custom infra, and you want time back to work on real product features, Samey is well worth a test drive—especially with usage-based pricing and meaningful startup credits. 🌟
If you already have mature infra, advanced compliance, or JVM-based ML, you might hit some edges early. But for most seed to A-stage founders, it lets your ML team spend less time as part-time DevOps—and more as value-creators.
Try the free plan. Run a pilot. Ask for credits. Match features to your roadmap—not the other way around. If Samey becomes a bottleneck, you can always step up to an open-source stack or enterprise-grade toolset later. For now? Get shipping—and let Samey do the ops heavy lifting for you. 🚀🔧